醫學圖像分析簡介
20世紀70年代,X線圖像在臨床廣泛套用,醫生藉助學習得到的知識和實踐積累的經驗,解讀病人的X線照片所反映的解剖結構和病生理信息。但是,這種人工解讀方式,往往依賴於醫生個人的經驗、知識和情緒,且效率較低。隨著計算機的發展和數位化儀器的出現,人們開始把X線膠片的模擬圖像轉變為數字圖像存儲和傳輸;另外,計算機技術的逐步成熟也提供了相當的計算能力。研究者開始嘗試把醫學模擬圖像轉化為數字圖像,開展了計算機輔助診斷(computer aided diagnosis,CAD)的初步研究,試圖在一定程度上輔助醫生判讀醫學圖像,排除人為主觀因素,提高診斷準確性和效率。
醫學臨床需求也推動著醫學圖像分析的發展。多排螺旋CT能夠在極短的時間內採集到真正的各向同性體素數據並重組成三維數據,MR心臟圖像是時間加三維的四維數據,一次完整的心臟檢查結果包括在心動周期上、各個時刻、多個層面上的數百張二維圖像。數據採集的高維化,導致依靠傳統的二維表達已經很難理解如此海量的信息,更談不上有效率地判讀和分析。醫學圖像分析的研究的對象也日益廣泛,不再局限於過去具有明顯診斷特徵的病種,開始擴展到多種不同器官、解剖形態、功能過程的圖像,試圖利用自動精確定量的計算機輔助圖像分析,幫助臨床醫生和研究者高效準確地處理海量圖像信息。在成像硬體的推動下,從20世紀90年代中期到現在,醫學圖像分析在理論方法和套用上都取得了長足的進步。
理論
分割
醫學圖像的感興趣區分割研究,是醫學圖像分析中最重要的基礎,準確、魯棒和快速的圖像分割,是定量分析、三維可視化等後續環節之前的最重要步驟,也為圖像引導手術、放療計畫和治療評估等重要臨床套用奠定了最根本的基礎。早期醫學圖像分割研究,主要是直接套用圖像處理的經典方法,如邊界提取和基於灰度的區域增長算法等。後來開始針對不同的解剖位置和成像模態,設計基於成像設備、成像參數和解剖結構等先驗知識的專用圖像分析算法,如利用多參數的MR圖像、決策樹和統計聚類技術來分割腦組織的白質和灰質。隨著圖像分析和計算機視覺理論方法的不斷豐富,醫學圖像感興趣區分割也出現了很多新突破。參數動態輪廓線模型,(active contour model)開創性地提出了高層模型指導、底層圖像特徵驅動的圖像分割思想,通過最小化模型的內外部能量得到分割結果,在隨後20多年中得到研究者的廣泛關注和不斷發展 。動態表現模型(active appearance model) ,動態形狀模型(active shape model) 都是這一類的代表方法。Osher和Sethian提出的水平集(1evel set)方法 ,利用數值方法模擬各種曲面演化,後來作為一種數值模擬和分析的方法,被廣泛運用於曲線或曲面的運動跟蹤,在醫學圖像分割中也得到廣泛套用。但目前大多數分割算法對醫學圖像成像參數和曲線、曲面的初始位置較敏感,沒有人工參與,難以做到魯棒分割不同設備乃至同種設備、不同參數的醫學圖像。
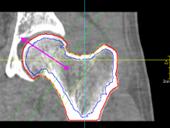
圖1. 醫學圖像分割
圖像配準和信息融合
早期醫學圖像的配準目的,是為了把顯示人體不同信息(結構信息和功能信息)的醫學圖像放到統一坐標系中顯示。隨著腦部結構形態學分析研究的進步,出現了同病人不同時期及同種疾病的病人群體圖像數據配準,以及標準圖譜到特定圖像數據集配準研究。為了消除成像過程中對象運動的影響,圖像配準也是時序圖像分析的第一步。尋找物體在不同影像中對應像素點的關係是醫學圖像配準的基本任務。通過尋找適當的空間變換,使圖像數據達到空間位置上的定位和配準,進而進行圖像融合。圖像配準方法可以分為基於外部特徵和基於內部特徵兩類。使用外部特徵的通過人工設定的標記點實現配準,如立體框架定位、皮膚標記法等;而基於內部特徵方法通過尋找圖像內部解剖結構上的特徵點或外部輪廓和表面的對應關係實現配準。互信息量是隨機變數統計相關性的測度,在醫學圖像配準中得到廣泛套用 。由於最大互信息方法不需要假設不同成像模式下的圖像灰度的相關性,也無需對圖像進行分割和預處理,非常適合三維多模醫學圖像配準,具有精度高、魯棒性強的特點。目前,醫學圖像配準技術和分割技術一樣,出現了很多成熟的方法和軟體,是醫學圖像分析中的基本技術之一。
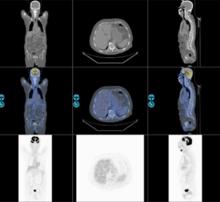
圖2. 醫學圖像配準融合
可視化
可視化(visualization)在醫學圖像分析中扮演重要角色。醫學圖像可視化技術是指利用從實驗中獲得的、掃描器測得的、計算模型合成的醫學圖像數據,重建三維圖像模型,並進行定性定量分析,為用戶提供具有真實感的三維醫學圖像,使人們更清楚地認識蘊涵在體數據中的複雜結構,便於醫生多角度多層次地觀察和分析,並且能夠使醫生有效參與數據處理與分析的過程。醫學圖像可視化技術,按照繪製過程中數據描述方法的不同,通常分成兩大類一面繪製和體繪製。面繪製技術是指對體表面重建,即從切片數據提供的三維數據場中抽取等值面,然後用傳統圖形學技術實現表面繪製。面繪製可以有效地繪製出物體表面,但缺乏對物體內部信息的表達。體繪製則是以體素作為基本單元,直接由切片數據生成三維物體圖像,表示物體內部信息,但是計算量很大。此外,將那些融合了這兩種技術特點的重建算法歸納為第3大類——混合繪製技術。
體繪製技術不需要構造中間對象,直接由三維數據本身來重建物體。三維數據中的各個數據作為一個表示實體的基本單元——體素,每個體素都有顏色、不透明度、梯度等相應屬性。體繪製的基本原理是模擬光線穿越半透明物質時能量的累積變化,即光線傳輸理論。具體說來,首先對每個體素賦以不透明度和顏色值(R、G、B);再根據各個體素所在點的梯度以及光照模型計算相應體素的光照強度;然後根據光照模型,將投射到圖像平面中同一個象素點的各個體素的不透明度和顏色值組合在一起,生成最終結果圖像。體繪製技術的典型算法有光線投影法、足跡法、錯切變形法、基於硬體的三維紋理映射、頻域體繪製法、基於小波的體繪製法等。
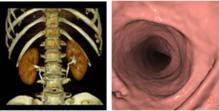
圖3. 醫學圖像可視化
時序圖像的功能分析
醫學圖像分析的目的是得到生理過程的定量信息,為臨床診療提供更充分的依據。過去的醫學成像主要集中在解剖結構成像和形態分析上。近年來,醫學設備成像速度的飛躍使對生理過程的動態觀察成為可能。例如:在腦功能成像領域,Functional MRI開始廣泛套用;核醫學PET(positron emission tomography)和SPECT(single photon emission computer tomography),在腦功能和心功能動態成像方面也得到廣泛的套用;MR心功能成像方面,標記MR技術(Tagging)和相位對比MR成像技術,在物理上為圖像定量分析心功能提供了依據;另外,三維超聲的出現,提供了實時觀察心室和瓣膜運動的手段。以上設備都能生成產生時序的二維或三維醫學圖像,反映了生理過程的動態功能信息。相應的醫學圖像分析也由圖像處理範疇的圖像分割、配準技術,向以醫學圖像為媒介,精確、定量地分析器官的功能和生理過程發展。
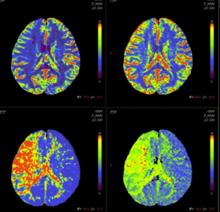
圖4. 腦部灌注分析
功能拓展及套用
基於內容的圖像檢索(content based image retrieval)在自然圖像和視頻檢索方面取得一定進步,通過提取輸入圖像的特徵,在圖像資料庫快速搜尋特徵空間中鄰近的類似圖像。隨著醫學數據的海量化趨勢加劇,近幾年來基於內容的醫學圖像檢索的研究與套用正形成熱點。圖像檢索系統可以幫助醫生在海量資料庫中快速尋找具有類似病理特徵並已確診的醫學圖像,提高疾病診斷的準確率。醫學圖像檢索有以下難點:首先,不同成像設備得到的醫學圖像內容特點差異巨大,很難用統一算法自動分析和提取特徵;其次醫學圖像的分類涉及專業知識,譬如區分不同種類的腦部腫瘤圖像,必須通過某種形式來結合專科影像學專業知識;再次,現代的醫學圖像多為三維或者更高維,這也給特徵提取和檢索算法提出了新的挑戰。上述困難使目前圖像檢索研究主要集中在針對特定成像設備和特定解剖位置,或疾病種類的醫學圖像。目前,大部分研究止於理論研究,只有及少數圖像檢索系統報告了臨床評估的表現和實際套用。
擴展閱讀
陳武凡, 秦安, 江少峰, 馮前進, 郝立巍. 醫學圖像分析的現狀與展望, 《中國生物醫學工程學報》,2008年第27卷第2期 175-181頁。
湯敏, 陳峰, 陶玲. 醫學圖像可視化及加速技術的研究進展和趨勢, 《中國組織工程研究與臨床康復》,2011年第15卷第4期 741-744頁。
J Gee, M Reivich, R Bajcsy (1993). "Elastically Deforming a Three-Dimensional Atlas to Match Anatomical Brain Images". Journal of Computer Assisted Tomography 17 (1): 225–236.
MR Sabuncu, BT Yeo, K Van Leemput, B Fischl, P Golland (June 2010). "A Generative Model for Image Segmentation Based on Label Fusion". IEEE Transactions on Medical Imaging 29 (10): 1714–1729.
Cootes TF, Taylor CJ, Cooper DH, and Graham J (1995). "Active shape models-their training and application". Computer vision and image understanding (San Diego: Academic Press, c1995-) 61 (1): 38–59.
Cootes, T.F. and Edwards, G.J. and Taylor, C.J. (2001). "Active appearance models". Pattern Analysis and Machine Intelligence, IEEE Transactions on (IEEE) 23 (6): 681–685.
R. Goldenberg, R. Kimmel, E. Rivlin, and M. Rudzsky (2001). "Fast geodesic active contours". IEEE Transactions on Image Processing 10 (10): 1467–1475.
Karasev, P., Kolesov I., Chudy, K. Vela, P., and Tannenbaum, A. (2011). "Interactive MRI segmentation with controlled active vision". Proceedings of the Conference on Decision and Control.
Lisa Gottesfeld Brown (1992). "A survey of image registration techniques". ACM Computing Surveys (CSUR) 24 (4): 325–376.
J. Maintz and M. Viergever (1998). "A survey of medical image registration". Medical Image Analysis 2 (1): 1–36.
J. Hajnal, D. Hawkes, and D. Hill (2001). Medical Image Registration. Baton Rouge, Florida: CRC Press.
Barbara Zitová, Jan Flusser (2003). "Image registration methods: a survey". Image Vision Comput. 21 (11): 977–1000.
J. P. W. Pluim, J. B. A. Maintz, and M. A. Viergever (2003). "Mutual information based registration of medical images: A survey". IEEE Trans. Med. Imaging 22 (8): 986–1004.
C. J. Twining and T. Cootes and S. Marsland and V. Petrovic and R. Schestowitz and C. Taylor. "A unified information-theoretic approach to groupwise non-rigid registration and model building". Proceedings of Information Processing in Medical Imaging 2005.
M. Sabuncu and S. K. Balci and M. E. Shenton and P. Golland (2009). "Image-driven Population Analysis through Mixture Modeling". IEEE Transactions in Medical Imaging 28 (9): 1473–1487.
K.J. Friston, W.D. Penny, C. Phillips, S.J. Kiebel, G. Hinton, and J. Ashburner (2002). "Classical and Bayesian Inference in Neuroimaging: Theory". NeuroImage 16 (2): 465–483.
Yong Fan, Nematollah Batmanghelich, Chris M. Clark, Christos Davatzikos, the Alzheimer’s Disease Neuroimaging Initiative, Spatial patterns of brain atrophy in MCI patients, identified via high-dimensional pattern classification, predict subsequent cognitive decline. (2008). "Spatial patterns of brain atrophy in MCI patients, identified via high-dimensional pattern classification, predict subsequent cognitive decline". NeuroImage 39 (4): 1731–1743.
Y. Wang and Y. Fan and P. Bhatt P and C. Davatzikos (2010). "High-dimensional pattern regression using machine learning: from medical images to continuous clinical variables". Neuroimage 50 (4): 1519–35.
Benoît Magnin and Lilia Mesrob and Serge Kinkingnéhun and Mélanie Pélégrini-Issac and Olivier Colliot and Marie Sarazin and Bruno Dubois and Stéphane Lehéricy and Habib Benali (2009). "Support vector machine-based classification of Alzheimer's disease from whole-brain anatomical MRI". Neuroradiology 51 (2): 73–83.
N.K. Batmanghelich and B. Taskar and C. Davatzikos (2012). "Generative-discriminative basis learning for medical imaging". IEEE Trans Med Imaging 31 (1): 51–69.
Glenn Fung and Jonathan Stoeckel (2007). "SVM feature selection for classification of SPECT images of Alzheimer's disease using spatial information". Knowledge and Information Systems 11 (2): 243–258.
1.陳武凡, 秦安, 江少峰, 馮前進, 郝立巍. 醫學圖像分析的現狀與展望, 《中國生物醫學工程學報》,2008年第27卷第2期 175-181頁。
2.湯敏, 陳峰, 陶玲. 醫學圖像可視化及加速技術的研究進展和趨勢, 《中國組織工程研究與臨床康復》,2011年第15卷第4期 741-744頁。
3.J Gee, M Reivich, R Bajcsy (1993). "Elastically Deforming a Three-Dimensional Atlas to Match Anatomical Brain Images". Journal of Computer Assisted Tomography 17 (1): 225–236.
4.MR Sabuncu, BT Yeo, K Van Leemput, B Fischl, P Golland (June 2010). "A Generative Model for Image Segmentation Based on Label Fusion". IEEE Transactions on Medical Imaging 29 (10): 1714–1729.
5.Cootes TF, Taylor CJ, Cooper DH, and Graham J (1995). "Active shape models-their training and application". Computer vision and image understanding (San Diego: Academic Press, c1995-) 61 (1): 38–59.
6.Cootes, T.F. and Edwards, G.J. and Taylor, C.J. (2001). "Active appearance models". Pattern Analysis and Machine Intelligence, IEEE Transactions on (IEEE) 23 (6): 681–685.
7.R. Goldenberg, R. Kimmel, E. Rivlin, and M. Rudzsky (2001). "Fast geodesic active contours". IEEE Transactions on Image Processing 10 (10): 1467–1475.
8.Karasev, P., Kolesov I., Chudy, K. Vela, P., and Tannenbaum, A. (2011). "Interactive MRI segmentation with controlled active vision". Proceedings of the Conference on Decision and Control.
9.Lisa Gottesfeld Brown (1992). "A survey of image registration techniques". ACM Computing Surveys (CSUR) 24 (4): 325–376.
10.J. Maintz and M. Viergever (1998). "A survey of medical image registration". Medical Image Analysis 2 (1): 1–36.
11.J. Hajnal, D. Hawkes, and D. Hill (2001). Medical Image Registration. Baton Rouge, Florida: CRC Press.
12.Barbara Zitová, Jan Flusser (2003). "Image registration methods: a survey". Image Vision Comput. 21 (11): 977–1000.
13.J. P. W. Pluim, J. B. A. Maintz, and M. A. Viergever (2003). "Mutual information based registration of medical images: A survey". IEEE Trans. Med. Imaging 22 (8): 986–1004.
14.C. J. Twining and T. Cootes and S. Marsland and V. Petrovic and R. Schestowitz and C. Taylor. "A unified information-theoretic approach to groupwise non-rigid registration and model building". Proceedings of Information Processing in Medical Imaging 2005.
15.M. Sabuncu and S. K. Balci and M. E. Shenton and P. Golland (2009). "Image-driven Population Analysis through Mixture Modeling". IEEE Transactions in Medical Imaging 28 (9): 1473–1487.
16.K.J. Friston, W.D. Penny, C. Phillips, S.J. Kiebel, G. Hinton, and J. Ashburner (2002). "Classical and Bayesian Inference in Neuroimaging: Theory". NeuroImage 16 (2): 465–483.
17.Yong Fan, Nematollah Batmanghelich, Chris M. Clark, Christos Davatzikos, the Alzheimer’s Disease Neuroimaging Initiative, Spatial patterns of brain atrophy in MCI patients, identified via high-dimensional pattern classification, predict subsequent cognitive decline. (2008). "Spatial patterns of brain atrophy in MCI patients, identified via high-dimensional pattern classification, predict subsequent cognitive decline". NeuroImage 39 (4): 1731–1743.
18.Y. Wang and Y. Fan and P. Bhatt P and C. Davatzikos (2010). "High-dimensional pattern regression using machine learning: from medical images to continuous clinical variables". Neuroimage 50 (4): 1519–35.
19.Benoît Magnin and Lilia Mesrob and Serge Kinkingnéhun and Mélanie Pélégrini-Issac and Olivier Colliot and Marie Sarazin and Bruno Dubois and Stéphane Lehéricy and Habib Benali (2009). "Support vector machine-based classification of Alzheimer's disease from whole-brain anatomical MRI". Neuroradiology 51 (2): 73–83.
20.N.K. Batmanghelich and B. Taskar and C. Davatzikos (2012). "Generative-discriminative basis learning for medical imaging". IEEE Trans Med Imaging 31 (1): 51–69.
21.Glenn Fung and Jonathan Stoeckel (2007). "SVM feature selection for classification of SPECT images of Alzheimer's disease using spatial information". Knowledge and Information Systems 11 (2): 243–258.